Your AI Proof of Concept
So, you’ve begun your Artificial Intelligence (AI) or Machine Learning (ML) journey and executed a project that demonstrates a valid return on investment (ROI). All of your important stakeholders have bought in, and now you want to deploy this proof of concept (PoC) across the entire organization – what is typically called “enterprising”. Some questions you may be asking yourself: How far are we from deploying this solution for the entire business group? Do we know where the gaps are?
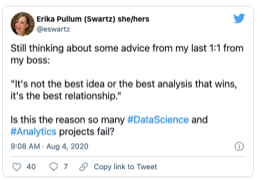
The data space vs software engineering
While data has been around a “long long” time, data volumes have recently been accelerating at an exponential rate and have outpaced the scaling of the software engineering related to data. While traditional software has adapted and become more complex, it has benefited from the time to mature in both function and approach. Agile development, testing, continuous integration /continuous development (CI/CD), and proper project management are approaches and tools that have made enterprise software delivery possible.
However, the data space is much more nuanced because AI and ML involve both the functional aspects associated with software engineering development, as well as the robust content derived from data Like software development, the AI and ML space has also adopted agile development and Continuous Integration and Deployment (CI/CD), but given the scale of data is challenged when it comes to testing and data quality. testing includes testing for quality, which means that testing a subset of data often is not enough. AIL initiatives require large amounts of good quality data which is often an issue at the PoC stage because it has simply not been collected. This will become an even larger issue as the initiative is scaled. Generally, data quality is less of a challenge at the PoC stage, as the data volumes are smaller, and the initiative is time boxed.
Arcurve is enterprise software
Arcurve was born with the intention that there is a better way to create software. Mature project management, agile development, and use of tools carefully selected for each client have helped Arcurve deploy enterprise software for a variety of clients, large and small. The Arcurve methodology means that the riskier components of the project are executed first, usually in the proof of concept (PoC) stage. Tailing risks can be managed in the next stage of the project, where it is converted to a minimum viable product (MVP) which de-risks the project for the final stage when it is taken into full production.

Learning has more to do with the problem being solved, rather than the technology. In the PoC phase, everyone can learn if and how the problem can be solved. Learning continues in the MVP, as everyone better understands how users interact with the software. Learning eventually tapers off as we understand how users interact with the software, and how it performs.
Arcurve is also enterprise AI
Arcurve Advanced Analytics helps clients build enterprise AI with the same maturity we deliver software. Project management, agile delivery, carefully selected tools, and a wholistic approach help clients realize the value of their enterprise AI initiatives while managing the risk through the entire process.

The same framework for enterprise software development is applicable to enterprise AI. Many of the pillars are like the steps required in software engineering; it is worthwhile addressing each step discretely, as each one has explicit data driven nuances.

So, where are the stumbling blocks when enterprising AI?
Here are the five enterprise AI pillars that that we consider critical when enterprising AI:
1. Wholistic approach - Understanding the big picture is critical. The ability to see and measure the ROI of your initiative across the business, helps you keep a focus on maintaining thatROI. Often, an optimization initiative may have an adverse effect such as creating additional costs or other unintended consequences elsewhere in the business.
2. Digital data infrastructure – Collecting all your data in one place is foundational.. This pillar must be managed strategically because not all initiatives will be managed the same way. If data infrastructure is not set up strategically, the initiative may end up with an architecture bespoke to the initial solution. Inevitably, different data points will be required when the initiative is in production, and care must be taken to ensure that the foundational sections can accommodate the scaling of the project.
3. Data quality - There are two drivers for data quality that can change over time:Physical sensors on machinery or facilities can drift; it may look like they are still working but are reporting incorrect values. The second driver is that processes and people upstream of that data change, and data quality can erode. Adequate reporting, alerts, and notifications can help you address these challenges before trust is lost and data quality deteriorates.
4. Trust – Many enterprise AI systems still require people which may then introduce human errors. It must be recognized that it is often times difficult to build trust into AI initiatives in the first place, as many people feel it threatens their employment. In fact, the initiative should be positioned as human augmentation rather than human replacement.
5. Managing change – As your business changes, the physical assets as well as the skilled personnel often change. Sensors being replaced may create “havoc” in machine learning models where sensors have been explicitly refreshed. Attrition among data scientists, data engineers, and Machine Learning Operations skillsets are highly sought after, and may result in turnover. Enterprise AI is truly enterprise if it can be supported by different team members in your organization.